Approach
- Materials data are often fragmented across multiple PDFs or databases. Our group assembles useful data into machine-readable datasets for our use and for public dissemination.
- The group focuses on providing physical understanding of materials systems with complex interactions. We therefore prioritize tools from supervised and unsupervised machine learning that supply interpretable results when used.
- Linear models and tree-based methods have straightforward feature importances that highlight dominant distortions when searching for a structure-property relationship.
- Dimensionality reduction techniques like principal component analysis and uniform manifold approximation and projection can map multiple features into a latent space where clusters can be distinguished.
- The ultimate goal of the informatic system is to enhance the capabilities of the researcher, not replace them.
Selected Publications
Crystal-Chemistry Guidelines for Noncentrosymmetric A2BO4 Ruddlesden−Popper Oxides
Noncentrosymmetric (NCS) phases are seldom seen in layered A2BO4 Ruddlesden−Popper (214 RP) oxides. In this work, we uncovered the underlying crystallographic symmetry restrictions that enforce the spatial parity operation of inversion and then subsequently showed how to lift them to achieve NCS structures. Simple octahedral distortions alone, while impacting the electronic and magnetic properties, are insufficient. We showed using group theory that the condensation of two distortion modes, which describe suitable symmetry unique octahedral distortions or a combination of a single octahedral distortion with a “compositional” A or B cation ordering mode, is able to transform the centrosymmetric aristotype into a NCS structure. With these symmetry guidelines, we formulated a data-driven model founded on Bayesian inference that allowed us to rationally search for combinations of A- and B-site elements satisfying the inversion symmetry lifting criterion. We described the general methodology and applied it to 214 iridates with A2+ cations, identifying RPstructured Ca2IrO4 as a potential NCS oxide, which we evaluated with density functional theory. We found a strong energetic competition between two closely related polar and nonpolar low-energy crystal structures in Ca2IrO4 and suggested pathways to stabilize the NCS structure.

Figure: Posterior probabilities of mode distortion given a specific (a) A-site or (b) B-site element
Learning from data to design functional materials without inversion symmetry
Accelerating the search for functional materials is a challenging problem. We developed an informatics-guided ab initio approach to accelerate the design and discovery of noncentrosymmetric materials. The workflow integrated group theory, informatics and density-functional theory to uncover design guidelines for predicting noncentrosymmetric compounds, which we applied to layered Ruddlesden-Popper oxides. Group theory identified how configurations of oxygen octahedral rotation patterns, ordered cation arrangements and their interplay break inversion symmetry, while informatics tools learned from available data to select candidate compositions that fulfil the group-theoretical postulates. Our key outcome was the identification of 242 compositions after screening ∼3,200 that show potential for noncentrosymmetric structures, a 25-fold increase in the projected number of known noncentrosymmetric Ruddlesden-Popper oxides. We validated our predictions for 19 compounds using phonon calculations, among which 17 had noncentrosymmetric ground states including two potential multiferroics. Our approach enables rational design of materials with targeted crystal symmetries and functionalities.
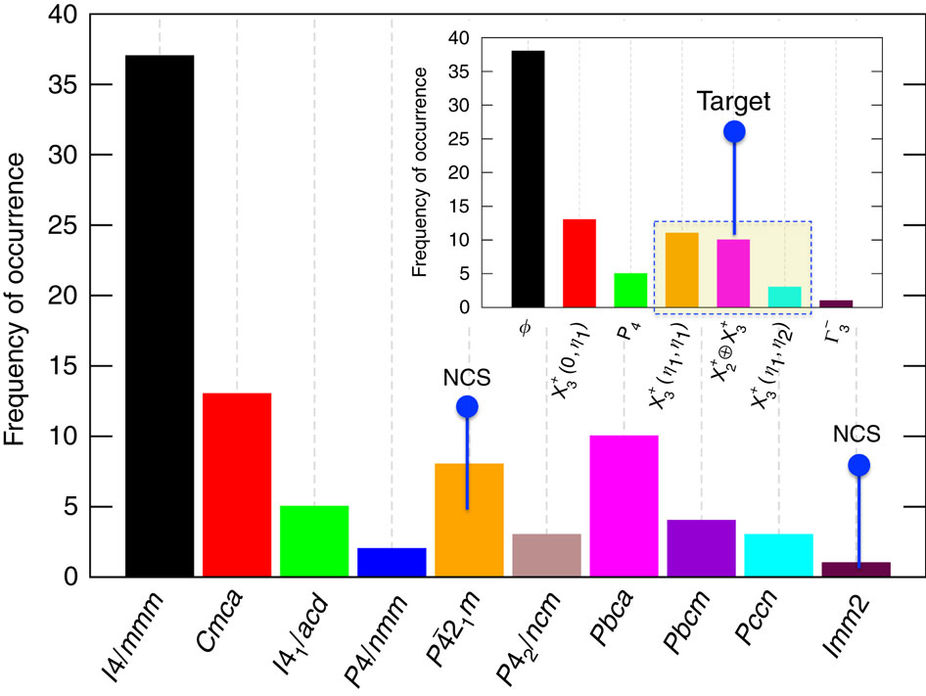
Figure: Distribution of experimentally known RP oxides.
Our survey of existing experimental reports resulted in a total of 84 compounds, which represent only a small fraction of the overall combinations of hypothetically feasible chemistries. Except for the nine compounds indicated in space groups P21m and Imm2, there were no other experimental reports of NCS phases in n=1 RP oxides.
Materials Prediction via Classification Learning
In the paradigm of materials informatics for accelerated materials discovery, the choice of feature set (i.e. attributes that capture aspects of structure, chemistry and/or bonding) is critical. Ideally, the feature sets should provide a simple physical basis for extracting major structural and chemical trends and furthermore, enable rapid predictions of new material chemistries. Orbital radii calculated from model pseudopotential fits to spectroscopic data are potential candidates to satisfy these conditions. Although these radii (and their linear combinations) have been utilized in the past, their functional forms are largely justified with heuristic arguments. We showed that machine learning methods naturally uncover the functional forms that mimic most frequently used features in the literature, thereby providing a mathematical basis for feature set construction without a prioriassumptions. We apply these principles to study two broad materials classes: (i) wide band gap AB compounds and (ii) rare earth-main group RM intermetallics. The AB compounds serve as a prototypical example to demonstrate our approach, whereas the RM intermetallics show how these concepts can be used to rapidly design new ductile materials. Our predictive models indicate that ScCo, ScIr, and YCd should be ductile, whereas each was previously proposed to be brittle.
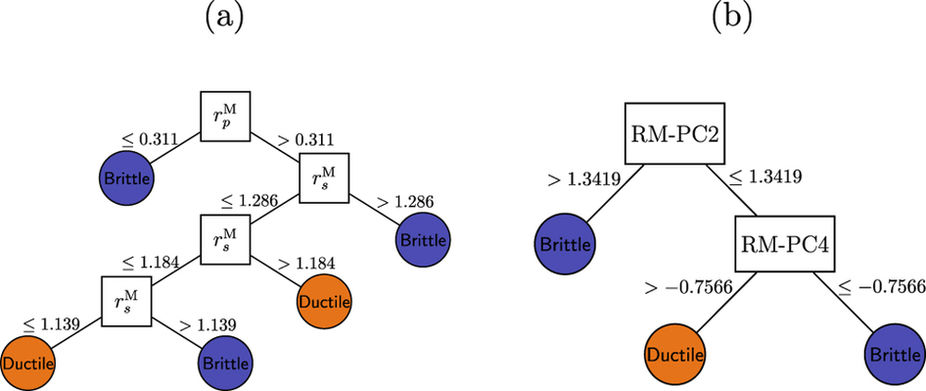
Figure: Decision trees for classifying ductile and brittle B2 RM intermetallics based on the full training set.
(a) Using raw Waber-Cromer orbital radii data and (b) after PCA to obtain their linear combinations. We found reduced dimensional forms of the Waber-Cromer orbital radii through principal component analysis that were as accurate at predicting ductility as quantities derived from theory. Thus, we provided an example where statistical learning was effective at capturing the same information discovered by expert physicists.